Chasing AI
By Rick Chavie
Founder and CEO, Firefly Kinetics
Democratization of AI
AI’s potential makes for a decision that all businesses will have to face: how do I surf this latest wave of creative destruction? Offload routine work to one of the many generative AI toolsets? Tackle complex, high-value decisions with AI where other business intelligence tools are lacking? Bring expensive talent in-house to plot a path with AI or rely on external experts?
AI tools are now commonplace: tools based on large language models (LLMs) such as ChatGPT (and its derivative in Microsoft’s Bing) or Jasper AI and HubSpot for marketing; generative AI e-commerce tools from Amazon for producing product descriptions and eBay for content derived from characterizing photos; Shopify and Adobe with multipronged GenAI for e-commerce and marketing; and Bard from Google’s which also leverages a user’s account data.
More, Better Boilerplate
Given the pervasiveness of AI offerings, major companies are making investments in AI, with three in four business leaders rating generative AI (GenAI) as the top emerging technology. These leaders see the top value for AI in improving efficiencies, with 97% of leaders agreeing that deploying GenAI AI will allow employees to be more thoughtful and creative by relieving them from manual processes.1
But seriously? A consensus goal of AI use focuses on efficiency improvements for routine manual processes, e.g., better boilerplate content. However, this consensus choice of GenAI for routine use in content generation also risks LLM “errors with confidence” (or “hallucinations”). OpenAI’s CEO Sam Altman acknowledges these quality issues associated with LLMs: “I probably trust the answers that come out of ChatGPT the least of anybody on Earth.”2
Commerce Complexity
As digitalized commerce continues to expand, along with AI-generated digital ad clutter, marketers face rising complexity in personalizing offers to consumers. Among the key issues:
- The proliferation of touchpoints: social media, texts, mobile, short-form video, QR codes, email, direct mail, websites, kiosks, POS, Smart Voice Assistants (SVA), etc.
- Overcoming the deprecation of third-party cookies, upon which 85% of them have relied to identify and track target customers across commerce touchpoints.3
- Identifying the permutations of product attributes preferred by individual consumers.
- Measuring consumer behavior across digital and physical channels.
For marketers, the complexities do not stop there as they look to AI: “It turns out there’s a fatal flaw in most companies’ approach to machine learning, the analytical tool of the future: 87% of projects do not get past the experiment phase and so never make it into production.”4
The resources and priority companies are placing on GenAI efficiency initiatives will improve the odds of getting to production and taking out costs. However, using AI for scalable demand generation that is differentiable from competitors (with the AI same tools) is a higher hurdle.
Models Breaking Badly
Marketers are creative in evolving 1:1 or persona-based personalization models, turning the “flawed” creativity of GenAI into a source for new ideas and finding new ways to track consumers in the cookieless commerce era. With the deprecation of third-party data, they now embrace zero-party data (e.g., direct consumer surveys), first-party data from their own websites (own cookies can still be used), and second-party data from partner or broker sources.
76% of marketers do not use behavioral data for online ad targeting. Measuring and using behavior data proves to be incredibly difficult for marketers. For example, only 13% of marketers said that their companies are prioritizing segmented experiences and measuring results. The State of Marketing Trends in 2023, from AI to Z, HubSpot
The increased effort to procure and synchronize these new data sources in service of 1:1 personalization suggests that the use of personas (i.e., representative customer segments typically based on demographic, psychographic, and even biometric data) will be a preferential strategy for aligning customized AI content and offers to segments.
Soon, even the persona approach may be a challenge: “90% of online content will be synthetically generated by the end of the decade,” maintains Nina Schick, author of Deepfakes: The Coming Infocalypse. What could go wrong in marketing to fictional individuals (personas) with generative technology that is trained on legacy and synthetic LLM training data and is prone to hallucinations? And in responding to changes in highly dynamic digitalized markets where competition and new products emerge frequently and are just a click away?
How good will legacy LLM data prove to be in the case of consumer products where an average of 30,000 new products are launched each year? In grocery, 57% of consumers surveyed say they purchased a new product during their last grocery shopping trip. NielsenIQ
Chasing Perfection Routinely
Vince Lombardi of the Green Bay Packers said, “Perfection is not attainable, but if we chase perfection, we can catch excellence.” Perfection in this case is avoiding the pitfalls of AI while mining the amazing potential. But excellence in AI should lift both the top and bottom lines to differentiate and grow in competitive, digitally enabled markets.
Observation: Game-changing upsides will come from using AI to change our decisions: replace mediocre choices with routinely better decisions and content having predictably better outcomes. Successful structuring of AI use can move company productivity to the right of today’s bell curve of outcomes. Not perfect outcomes, but ones that routinely accelerate market share gains.
The following are the keys to achieving AI success:
- Implementing guardrails for GenAI. The guardrails come in several forms, including “priming the pump” with concepts to keep it on message, forcing certain precise content into the output (e.g., for a product description, there can be legal liability if content inaccurately describes food ingredients, pharma instructions, etc.)
- For LLM applications, incorporate internal training data in producing content that is better aligned with your brand and your customers. This training data should include touchpoints to better pinpoint how and when customers receive offers and shop.
- Incorporate collaborative approaches with AI tools to unite their processing power with human creativity. Such collaboration produces greater results than either alone. In the book, the Second Machine Age by MIT’s Erik Brynjolfsson and Andrew McAfee, the authors make a compelling case for the superior outcomes of such an approach
- Don’t be satisfied with efficiencies. Beyond the obsession with content generation, AI-enabled decision-making for demand generation offers great upside. Lifting marketers, product managers, and sales teams from average to good or excellent is in play with these technologies and should be pursued aggressively to differentiate a company’s outcomes.
- Deploy “atomic level” measurement of results. Systems today can process enormous amounts of data yet effective measurement requires investing in high-quality data. Great granular data with off-the-shelf AI can outperform a great AI tool with poor data.
- Massively scale test and learn. “Black box” AI obscures insight into the “how” of results, much less attribution to specific marketing tactics. Yet, precise measurement of changes in variables, such as which touchpoint is used and when – or altering specific words - in offer design, can divine the “why” with robust feedback loops in live testing.
So, enjoy the chase on your way to AI excellence!
[1] KPMG Generative AI Survey: From buzz to business value. June 2023 update of 200 US business executives across industries in organizations with $1 billion or more in revenue on their views of generative AI.
[2] Tech experts are starting to doubt that ChatGPT and A.I. ‘hallucinations’ will ever go away. Fortune, Matt O’Brien, and the AP. August 1, 2023
[3] The State of Marketing Trends in 2023, from AI to Z. HubSpot.
[4] 35+ Amazing Advertising Statistics [2023]: Data + Trends. Jack Flynn. Jun. 13, 2023
[4] Why do 87% of data science projects never make it into production? Venture Beat, July 19, 2019
[Blockquote 2] Bursting with new products, there has never been a better time for breakthrough innovation. NielsenIQ. Dec 5, 2019
[Blockquote 2] Looking to achieve new product success? NielsenIQ. 23 June 2015
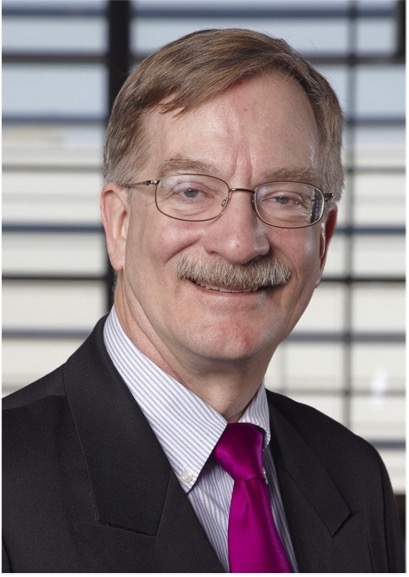
About the author
Rick Chavie embraces a holistic approach to business innovation. In addition to having been the CEO of InfoLenz, an AI company, he (1) led the turnaround and highly successful exit of EnterWorks (now part of Precisely) as CEO of this software provider, taking it from obscurity to global Leader status in MDM and PIM in the Forrester Waves, (2) collaborated with the founders of Home Depot and later CEOs to scale their already successful business: led the redesign and roll out of a new store model and installed advanced enterprise and digital technologies, (3) reimagined SAP’s B2C and B2B verticals: led their global retail and wholesale units and was the catalyst for SAP to acquire Hybris, the leading e-commerce software company where he was the Chief Solution Officer. Rick is a Harvard MBA, a Fulbright Scholar in International Trade, a Mentor at MIT’s Sandbox Innovation Fund, speaks fluent German, and loves to play tennis against tough competition.
By Rick Chavie
Founder and CEO, Firefly Kinetics
Democratization of AI
AI’s potential makes for a decision that all businesses will have to face: how do I surf this latest wave of creative destruction? Offload routine work to one of the many generative AI toolsets? Tackle complex, high-value decisions with AI where other business intelligence tools are lacking? Bring expensive talent in-house to plot a path with AI or rely on external experts?
AI tools are now commonplace: tools based on large language models (LLMs) such as ChatGPT (and its derivative in Microsoft’s Bing) or Jasper AI and HubSpot for marketing; generative AI e-commerce tools from Amazon for producing product descriptions and eBay for content derived from characterizing photos; Shopify and Adobe with multipronged GenAI for e-commerce and marketing; and Bard from Google’s which also leverages a user’s account data.
More, Better Boilerplate
Given the pervasiveness of AI offerings, major companies are making investments in AI, with three in four business leaders rating generative AI (GenAI) as the top emerging technology. These leaders see the top value for AI in improving efficiencies, with 97% of leaders agreeing that deploying GenAI AI will allow employees to be more thoughtful and creative by relieving them from manual processes.1
But seriously? A consensus goal of AI use focuses on efficiency improvements for routine manual processes, e.g., better boilerplate content. However, this consensus choice of GenAI for routine use in content generation also risks LLM “errors with confidence” (or “hallucinations”). OpenAI’s CEO Sam Altman acknowledges these quality issues associated with LLMs: “I probably trust the answers that come out of ChatGPT the least of anybody on Earth.”2
Commerce Complexity
As digitalized commerce continues to expand, along with AI-generated digital ad clutter, marketers face rising complexity in personalizing offers to consumers. Among the key issues:
- The proliferation of touchpoints: social media, texts, mobile, short-form video, QR codes, email, direct mail, websites, kiosks, POS, Smart Voice Assistants (SVA), etc.
- Overcoming the deprecation of third-party cookies, upon which 85% of them have relied to identify and track target customers across commerce touchpoints.3
- Identifying the permutations of product attributes preferred by individual consumers.
- Measuring consumer behavior across digital and physical channels.
For marketers, the complexities do not stop there as they look to AI: “It turns out there’s a fatal flaw in most companies’ approach to machine learning, the analytical tool of the future: 87% of projects do not get past the experiment phase and so never make it into production.”4
The resources and priority companies are placing on GenAI efficiency initiatives will improve the odds of getting to production and taking out costs. However, using AI for scalable demand generation that is differentiable from competitors (with the AI same tools) is a higher hurdle.
Models Breaking Badly
Marketers are creative in evolving 1:1 or persona-based personalization models, turning the “flawed” creativity of GenAI into a source for new ideas and finding new ways to track consumers in the cookieless commerce era. With the deprecation of third-party data, they now embrace zero-party data (e.g., direct consumer surveys), first-party data from their own websites (own cookies can still be used), and second-party data from partner or broker sources.
The increased effort to procure and synchronize these new data sources in service of 1:1 personalization suggests that the use of personas (i.e., representative customer segments typically based on demographic, psychographic, and even biometric data) will be a preferential strategy for aligning customized AI content and offers to segments.
Soon, even the persona approach may be a challenge: “90% of online content will be synthetically generated by the end of the decade,” maintains Nina Schick, author of Deepfakes: The Coming Infocalypse. What could go wrong in marketing to fictional individuals (personas) with generative technology that is trained on legacy and synthetic LLM training data and is prone to hallucinations? And in responding to changes in highly dynamic digitalized markets where competition and new products emerge frequently and are just a click away?
Chasing Perfection Routinely
Vince Lombardi of the Green Bay Packers said, “Perfection is not attainable, but if we chase perfection, we can catch excellence.” Perfection in this case is avoiding the pitfalls of AI while mining the amazing potential. But excellence in AI should lift both the top and bottom lines to differentiate and grow in competitive, digitally enabled markets.
Observation: Game-changing upsides will come from using AI to change our decisions: replace mediocre choices with routinely better decisions and content having predictably better outcomes. Successful structuring of AI use can move company productivity to the right of today’s bell curve of outcomes. Not perfect outcomes, but ones that routinely accelerate market share gains.
The following are the keys to achieving AI success:
- Implementing guardrails for GenAI. The guardrails come in several forms, including “priming the pump” with concepts to keep it on message, forcing certain precise content into the output (e.g., for a product description, there can be legal liability if content inaccurately describes food ingredients, pharma instructions, etc.)
- For LLM applications, incorporate internal training data in producing content that is better aligned with your brand and your customers. This training data should include touchpoints to better pinpoint how and when customers receive offers and shop.
- Incorporate collaborative approaches with AI tools to unite their processing power with human creativity. Such collaboration produces greater results than either alone. In the book, the Second Machine Age by MIT’s Erik Brynjolfsson and Andrew McAfee, the authors make a compelling case for the superior outcomes of such an approach
- Don’t be satisfied with efficiencies. Beyond the obsession with content generation, AI-enabled decision-making for demand generation offers great upside. Lifting marketers, product managers, and sales teams from average to good or excellent is in play with these technologies and should be pursued aggressively to differentiate a company’s outcomes.
- Deploy “atomic level” measurement of results. Systems today can process enormous amounts of data yet effective measurement requires investing in high-quality data. Great granular data with off-the-shelf AI can outperform a great AI tool with poor data.
- Massively scale test and learn. “Black box” AI obscures insight into the “how” of results, much less attribution to specific marketing tactics. Yet, precise measurement of changes in variables, such as which touchpoint is used and when – or altering specific words - in offer design, can divine the “why” with robust feedback loops in live testing.
So, enjoy the chase on your way to AI excellence!
[1] KPMG Generative AI Survey: From buzz to business value. June 2023 update of 200 US business executives across industries in organizations with $1 billion or more in revenue on their views of generative AI.
[2] Tech experts are starting to doubt that ChatGPT and A.I. ‘hallucinations’ will ever go away. Fortune, Matt O’Brien, and the AP. August 1, 2023
[3] The State of Marketing Trends in 2023, from AI to Z. HubSpot.
[4] 35+ Amazing Advertising Statistics [2023]: Data + Trends. Jack Flynn. Jun. 13, 2023
[4] Why do 87% of data science projects never make it into production? Venture Beat, July 19, 2019
[Blockquote 2] Bursting with new products, there has never been a better time for breakthrough innovation. NielsenIQ. Dec 5, 2019
[Blockquote 2] Looking to achieve new product success? NielsenIQ. 23 June 2015